Gan Hands : Algorithmic Production
Liebig12, Berlin, 2018
From the dexterity of our hands we have built the technologies around us, from the first hand tools to the machines we utilize now. In these hands is something unique that not many other animals share, our hands are a symbol for what humans can produce.
As machine learning algorithms begin to generate artifacts that were thought to be uniquely in the human domain, such as literature, art and music, what is left for human's produce? Will the definition of what it means to be human have to change, to be something other than a producer? Particularly in a world where value is placed on production, indicative in the measurement of a country's worth coming from GDP, will we continue to value production or will we come to value something new?
Gan Hands is an installation that used a DCGAN built in Tensorflow to generate new “imagined” replications of human hands. A Deep Convolutional Generative Adversarial Network is a machine learning algorithm that takes in large amounts of data, in this case 11,000 images of hands, in order to learn a hierarchy of representations from the object parts. During it’s training period, the network begins to generates ideas of other possibilities based on the hierarchies it has learned, the visualizations going from blurry to more accurate or odd as the training unfolds.
LABOR
While the artifacts are creepy and eerie, inaccurate and accurate, the labor leading to this point is remarkable. From the original researchers putting out the first papers on GANs in 2014, to the physical computation hardware required (the training period utilized GPUs to parallel process all the computations, Paperspace was used to create a virtual machine somewhere on the continent of Europe to process all the data, exact server farm location unknown), to the days spent editing, formatting and aestheticizing the data, the labor and resources required for our algorithimically derived world is an interesting context to reflect on.
DATA
The dataset was partially printed in paper, so it was possible to see its size in physical space. This installation brought up questions of what could be made into data, binary points that can be made into math equations. For Pythagoras this was the world and all it’s contents, but is there some data that is easier to collect than others? And if water flows in the path of least resistance, and we continue to develop processes based on data, the question becomes will some things be highlighted and others left to become obsolete, unrecognized or unvalued. Where do emotions such as unconditional love, kindness and generosity fall in this equation?
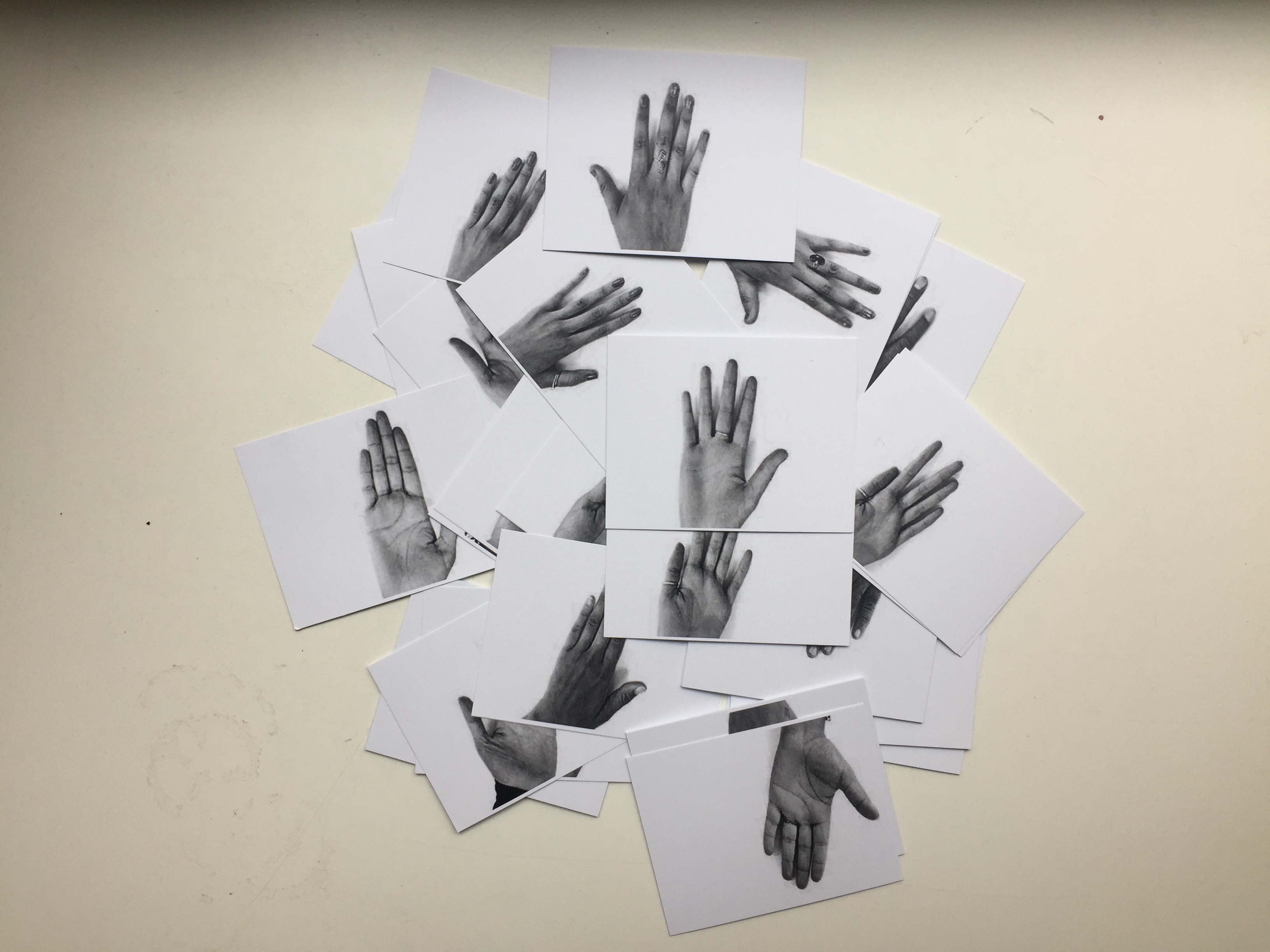
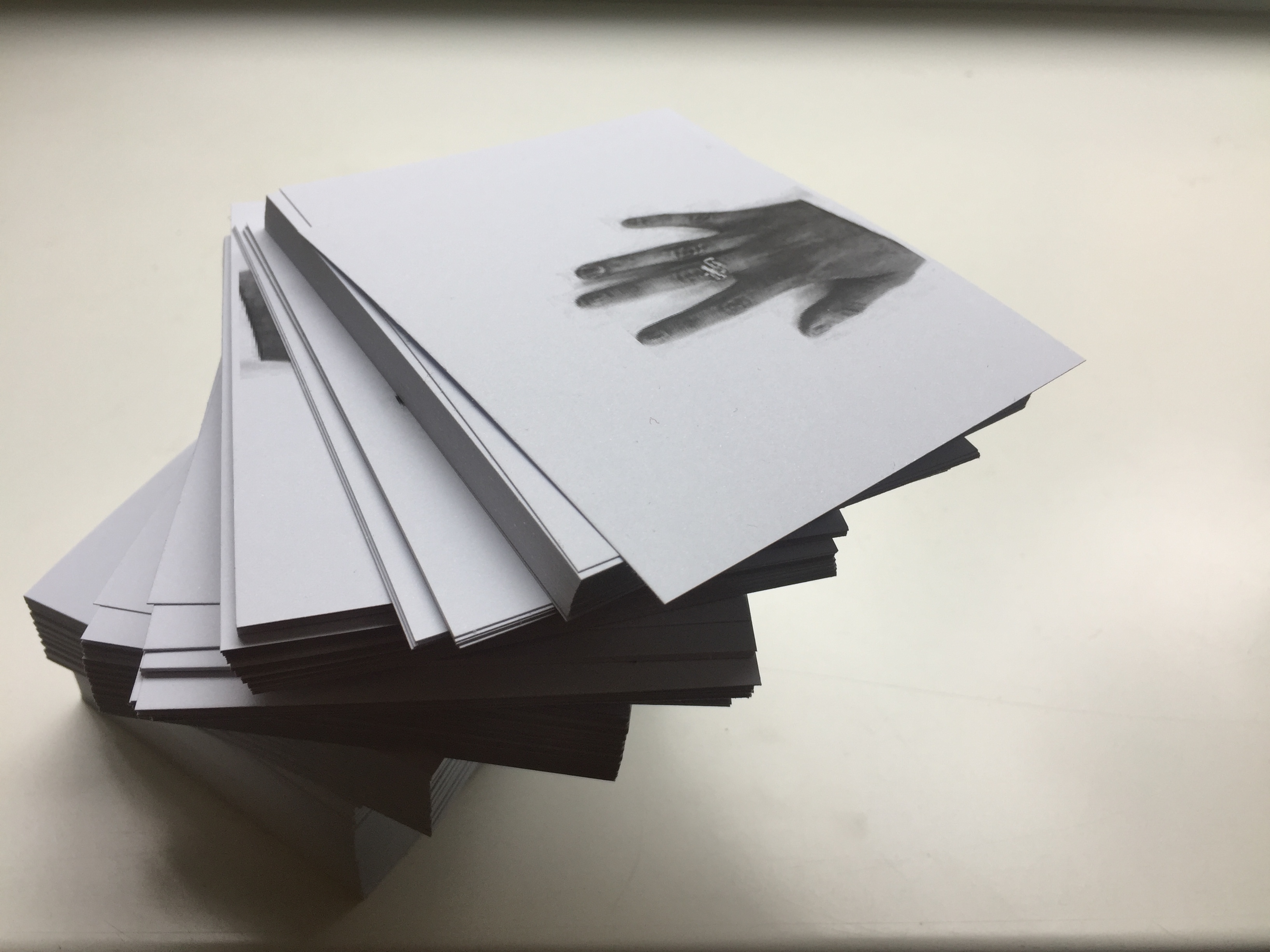
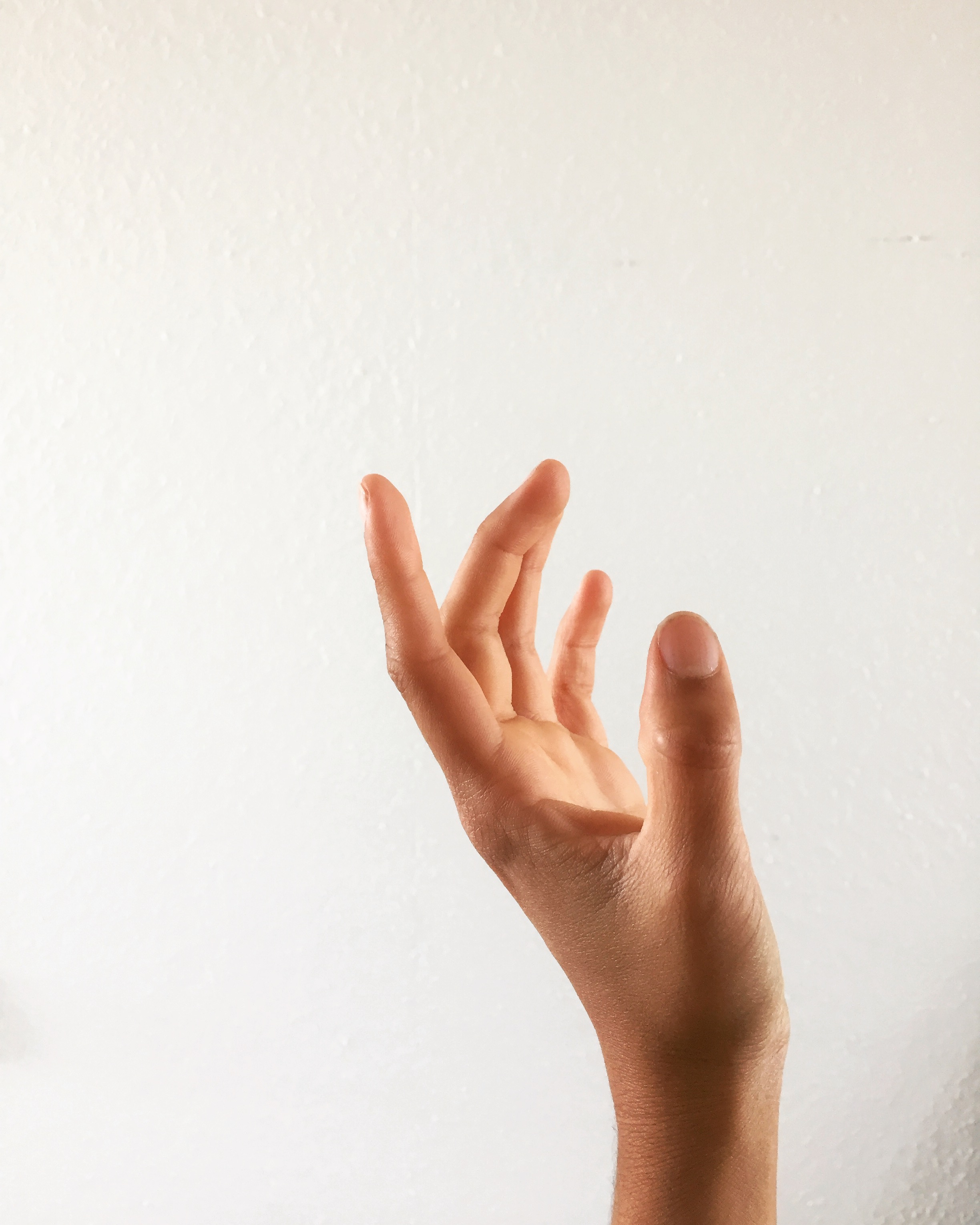
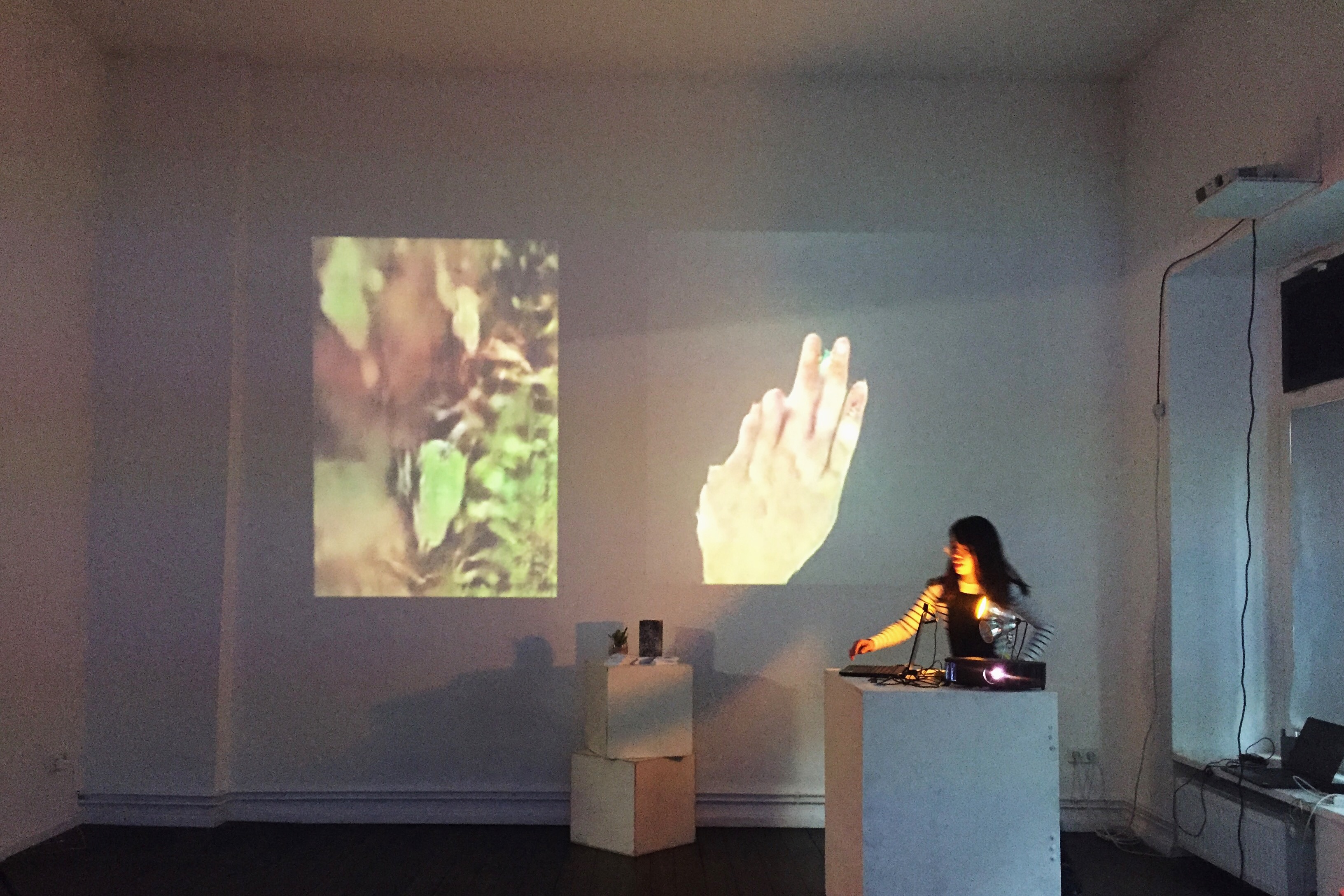
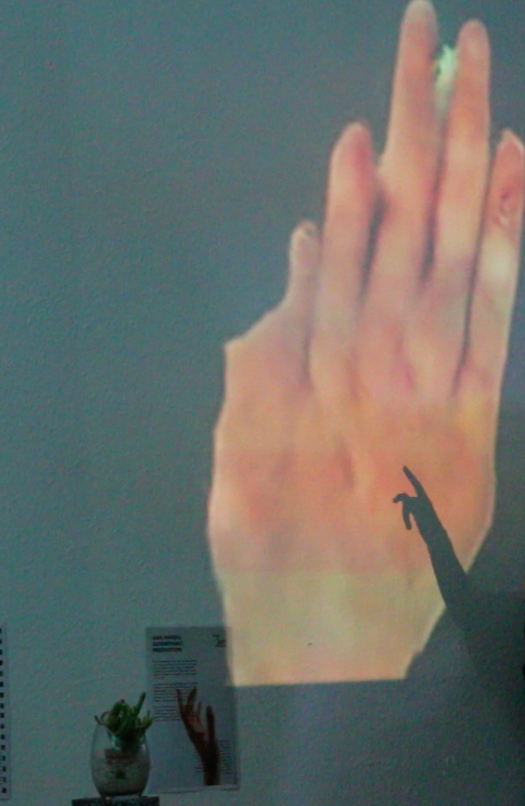